Chapter 1: The Promise of Industry 4.0 for the Production of Automotive Stampings and BiW Assemblies
The automotive industry is currently focusing heavily on digitalization to achieve massive automation technology improvements in productivity and production quality. This effort is in pursuit of the ultimate goal of creating an autonomous production environment – creating “smart factories”. Many different technologies, such as sensing technologies, artificial intelligence, Big Data, process automation, and IIoT (Industrial Internet of Things) technologies are being explored and evaluated in order to build the smart factory.
These visions are driven by the promise of many substantial benefits: self-correcting, intelligent production lines automatically making the necessary in-line process adjustments to ensure zero failure production (zero production scrap), maximum productivity and up-time (no unplanned production equipment down-times), lowest production cost and optimal production flexibility in order to be able to reduce lot sizes while maintaining profitability.
However, while a fully autonomous production environment will achieve much higher productivities and cost-effectiveness, it will not reduce development times and create higher flexibility of the design and development process to react to changing customer requirements and market trends.
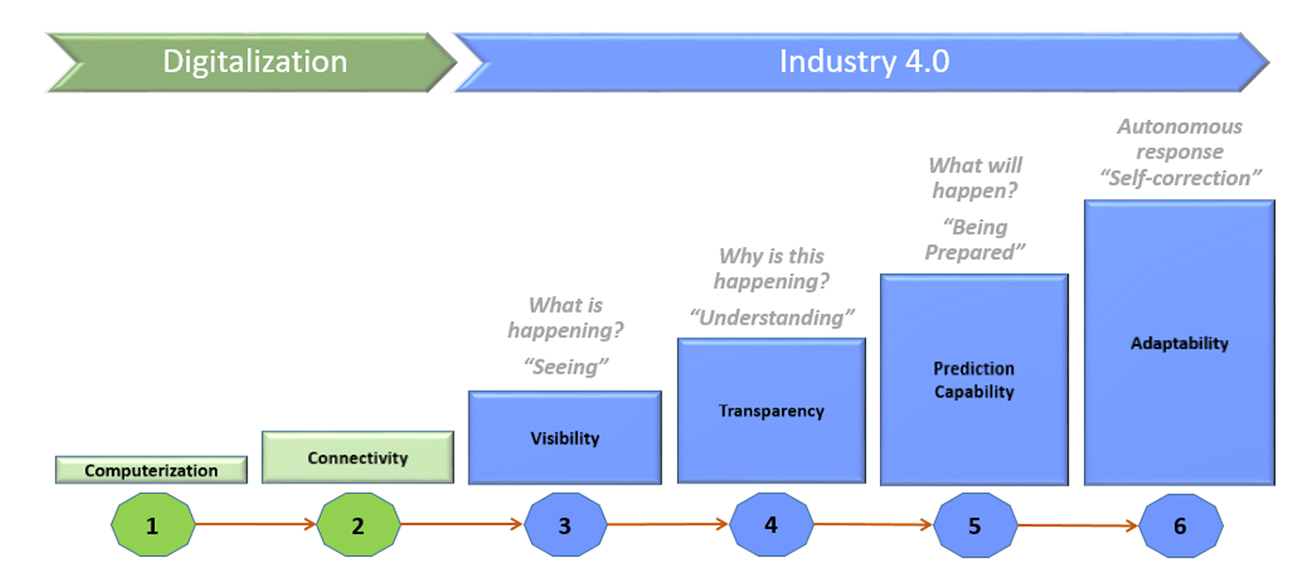
Fig. 1: Maturity Levels of Industry 4.0 acc. to Schuh et al. ([01] Günther Schuh)
The 6 maturity levels of Industry 4.0 – as published by the RWTH Aachen (see Figure 1) – give an indication that a lot of preparatory work needs to be done in order to achieve the highest level of “Autonomous Response”. There are some aspects of the process chain which today are not visible (digitally), some elements of the process chain are not digitally connected – and some activities, such as manual adjustments to a tool surface during the tool tryout process or inconsistent (disconnected) quality assurance systems in CAD/CAE/CAM processes, are not even computerized or connected.
So in order to aspire to the higher levels of Industry 4.0 implementation, work needs to be done on all levels following an overall strategy to implement digitalization strategies along the entire process chain involving all activities. This will need to be a joint effort of the entire automotive eco-system involving automotive companies themselves but also including all supply chains – for hardware, software, parts, sub-assemblies and services.
Industry 4.0 vs. the “Full Digitalization of the Stamping and BiW Assembly Process Chain”
While many concepts currently discussed in the context of Industry 4.0 focus on the production process, the “Full Digitalization” of the entire product and process creation takes a broader, more comprehensive view. Full digitalization not only means the use of advanced digital technologies to streamline and automate production processes, but also the comprehensive and integrated use of digital technologies in the entire product life cycle.
This includes product development, planning activities, process and production engineering as well as the digital support of the tool and fixture manufacturing, the actual tryout and ramp-up processes for stampings and assemblies as well as the production operations and quality management activities.
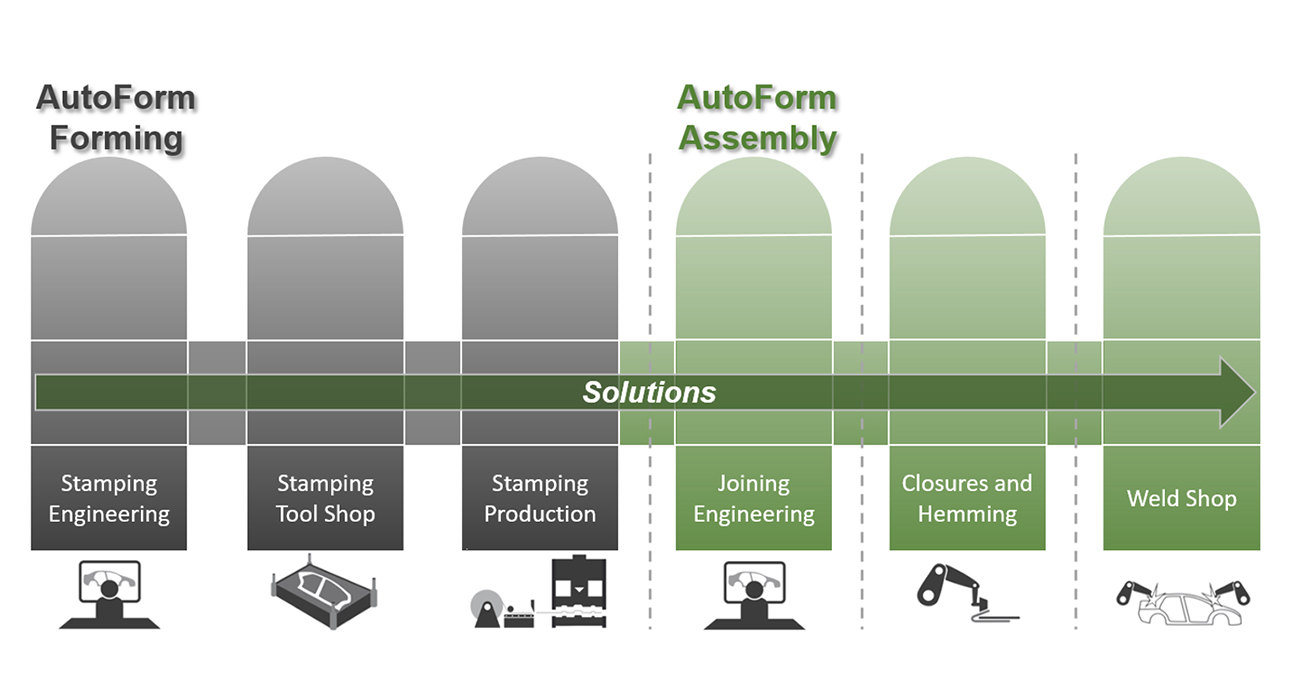
Fig. 2: Integrated BiW Process Chain [[02] Wiebenga], [[03] Ruggiero], ([04] Todd)
Full digitalization of the entire process chain promises even larger savings potentials than “just” the implementation of smart production strategies. A detailed analysis of typical OEM product development and creation processes shows that full digitalization of the entire stamping and BiW development process from inception until SOP may be shortened by approx. 6 to 9 months and that financial savings (CAPEX and OPEX) of over 10 Mio. Euro are achievable per vehicle project.
Fig. 3: Software tools for the integrated stamping
and BiW assembly process chain
The promise and the potentials are huge and many OEM’s have realized that their competitiveness in the coming decade will heavily depend on their advances regarding the digitalization of the entire product development process chain.
So – what do we mean by “Full Digitalization” of the automotive sheet metal product development process? And which aspects are of particular interest for those who want to progress toward that goal? What needs to be done – and in which order? These are the questions which are addressed – in the concrete context of the BiW and stamping process chains – in this paper.
In a nutshell: “Full Digitalization” means that all decisions and actions in the development and engineering process are supported and based on digital data, all activities in the process chain are digitally documented, searchable and transparent and that all stakeholders of the process – internally and externally – have full access to all relevant data describing the manufacturing process digitally.
Full Digitization
means that all decisions and actions in the development and engineering process are supported and based on digital data.
This may not sound terribly revolutionary since today most product development and engineering processes are performed with computers (i.e. digitally, CAD/CAE/CAM) already. However, if we begin to consider the details of what it means to truly bring all decision making processes to the digital level, we begin to realize that there is quite a distance to be covered.
To illustrate, let’s consider some examples of practices which are still being widely practiced by companies engaged in sheet metal forming, tool making and assembly operations worldwide:
These are some typical cases where the digital process chain may be interrupted and digitalization may fail. The list above is far from complete. However, the cases illustrate the fact that despite the widespread use of digital tools by product development and engineering, the path to a “full digitalization” remains long and winding.
The examples indicate that the major roadblock to a full digitalization is not a lack of digital tools for the existing process chain since for all issues described above, commercial and time-proven software solutions exist today. So what exactly is holding the digitalization of the process chain up?
The major roadblock
to full digitalization is not a lack of digitals tools.
The major reason why software usage in most automotive companies is not fully integrated into a fully digitalized supra-departmental process chain is the fact that each department – product development, planning and engineering, tool shop, press shop, BiW assembly, final assembly, paint shop, etc. – has done their best to support their activities with suitable software to perform their department-specific tasks with the highest effectiveness and efficiency. While this has brought great technological and commercial advances, it has naturally also led to very heterogeneous software landscapes making it hard to access, share and exchange engineering data with other departments and teams. This is the focus of the illustration in Figure 2 above.
With the exception of overarching data backbone systems such as ERP or PLM systems which are used throughout the organization, the engineering software systems used in the product development, engineering and production process have been limited in scope (e.g. Forming Simulation Software, MES, etc.).
With the current attention to Industry 4.0 topics, top management is now getting more involved and directs their attention to digitalization efforts in order to streamline the development process with the goal to reduce overall development investments and the time line.
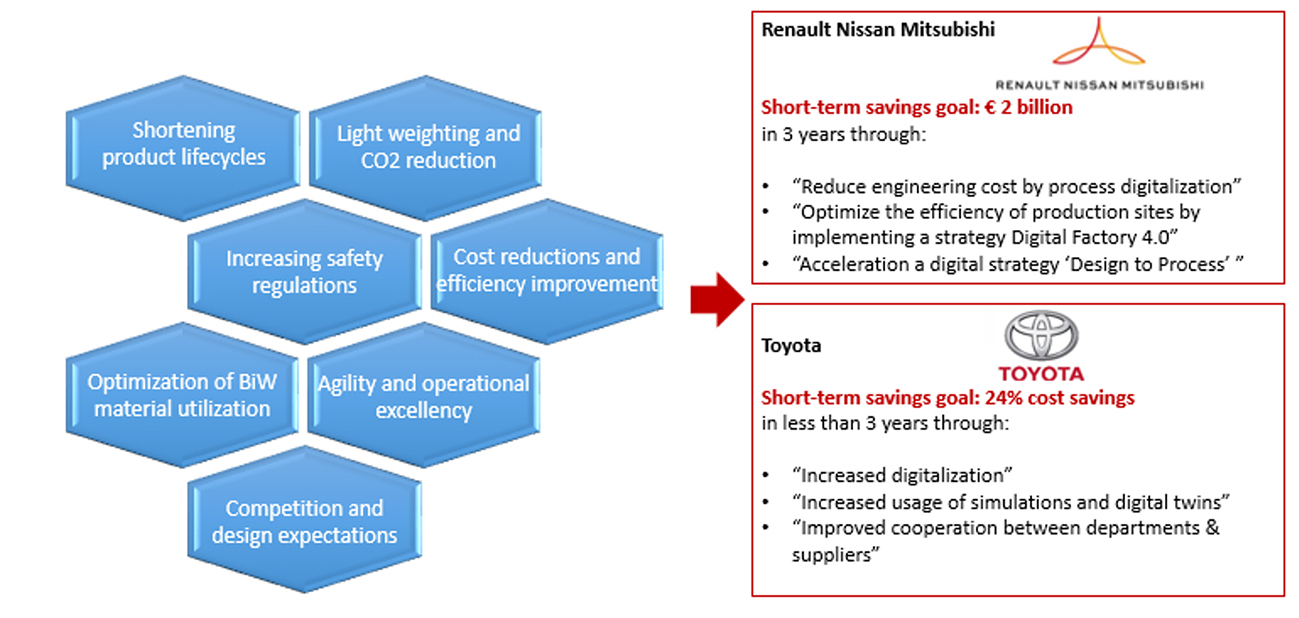
OEM Savings Goals Related to the Increased Use of Digital Technologies ([05] WiebengaJan)
However, while software and data continuity across stakeholding departments is a major issue, the actual process specifications are another. Development process specifications in many automotive companies, i.e. time lines, deadlines, milestones and standards, have not kept pace with the technological advances and do not reflect current best practices. This leads to a de-facto continuation of outdated practices and prevents the implementation of state-of-the-art digital analysis practices.
In summary, it is important to realize that the fully digitalized process chain will not be a “digital version” of the current process chain. But rather, it will be a different process chain altogether since important and useful data will become available much earlier, collaboration between teams and departments will become much more intimate and the decision making processes and timelines will need to be reconsidered. The full and exclusive reliance on digital data in the entire process chain means nothing short of a redesign of the entire process and the redefinition of the collaboration of all stakeholders inside this process.
The Fully Digitalized Process Chain
will not simply be a "digital version"
of the current process chain.
This paper’s focus is on presenting some important findings regarding the prerequisites and concepts which will help to start implementing such a digitalization process. AutoForm can assist this process not just with technology. The new digital process chain built around computer simulation as the core technology will have to focus on adjusting process and methodologies which are aligned with available state-of-the-art software technology. Some important aspects of this transformation will be discussed in this publication.
Chapter 2: Key Elements and Paradigms for Digitalization of the Stamping and BiW Assembly Process Chains
Smart Engineering and Smart Production
Clearly, the most effective and efficient path to “Smart Production” is leveraging the pre-existing engineering know-how to jump-start the production process. In modern automotive workflows a lot of human intelligence has already been invested into a production process before the first part is produced in a stamping press or a BiW assembly line. So why not leverage this know-how to accelerate production and ramp-up activities?
It seems obvious that in order to be of direct and immediate relevance for ramp-up and production, the engineering process needs to satisfy certain prerequisites and boundary conditions. How exactly can engineering activities help create and support “Smart Production” in this context? What are the indispensable ingredients to make process and production engineering “smart”?
Fig. 5: Blog publication on the importance of
process window assessment in digital process engineering
Keeping in mind that the major reason for “cutting corners” during the engineering process is not a lack of motivation or skill of the engineers – but rather that current development timelines often do not allow enough time to solve all issues in engineering – is an indication that the product development and engineering process will have to be reconsidered to systematically allow the implementation of smart engineering practices.
Fig. 6: Blog publication on the time line pressure in the
engineering process and their consequences ([06] Dorin ChertersMatrite)
The considerations listed above form some basic prerequisites for the engineering process to support “smart production” activities. In order to achieve a fully digitalized process chain – in pursuit of optimally efficient development processes leading up to a fully autonomous mass production environment – we need to take a more comprehensive view of the process and consider more than just “smart production” or “smart engineering”. These considerations are the subject of the next sections.
The Product Development and Engineering Process
will have to be reconsidered to systematically allow the implementation of smart engineering practices.
.
The Three Pillars of Digitalization in the Context of Manufacturing Simulation
As was already hinted at in the previous sections, a multi-dimensional approach needs to be taken when trying to fully digitalize the stamping and BiW assembly process chains.
Generally speaking, full digitalization needs the following three ingredients in order to be successful:
These three pillars “Software, Model and Process” may also be thought of as having correspondences regarding actions, namely verification, validation and implementation. While the software needs constant verification – which is predominantly executed during development by the software developper – the model building process and the resulting models need to be validated by users hand-in-hand with software vendors. The actual process changes reflecting the new digitalized process need to be implemented in the automotive process by automotive companies with assistance of software vendors.
In the following sections we will take a more detailed look at what exactly needs to be considered when trying to erect the three pillars described above.
Software Prerequisites to Support Industry 4.0 and the Fully Digitalized BiW Process Chain
The engineering analysis software used to create and analyze the computer model or the “digital twin” of the production process has to satisfy a number of prerequisites in order to allow its use for the full, i.e. complete, digitalization of the product development and production process.
Since the software system needs to support the entire product development and engineering process, “Digital Continuity” based on consistency of the digital data and digital tools is the most basic requirement. The software system must ensure full reusability and consistency of all data generated during the engineering process and hence, allow the use of the same data model used throughout development and engineering.
This also encompasses that the different modelling components – such as process plan, cost data, product data (part and/or BiW sub-assembly), engineering data – must be completely consistent with each other at all times. Or, at least, the inconsistency of data must be clearly and pro-actively indicated by the software system. The increasing degree of detail in different stages of the development process must be fully reflected by the data model and the interdepartmental flow of digital information must be fully supported. All elements of the planning, engineering and validation methodology must been fully aligned with internal manufacturing specifications and company standards. These specifications and standards must therefore be directly accessible and implemented in the software to ensure maximal compliance.
To support full digitalization, the software system must satisfy the following additional requirements:
AutoForm’s Market-Leading Offering for Implementation of Industry 4.0
AutoForm has done and is still doing everything to empower AutoForm customers to build a seamless digital process chain satisfying all of the above-mentioned requirements. This effort covers many different aspects in software development and testing procedures, product portfolio strategy, creation of best-practice procedures and methodologies as well as collaboration with customers and partners.
Software features for ensuring consistency and high modelling quality:
Software features ensuring easy access to data and results:
Testing procedures ensuring verification and consistency of all software modules and features with each other:
Broad process coverage thru a complete product portfolio
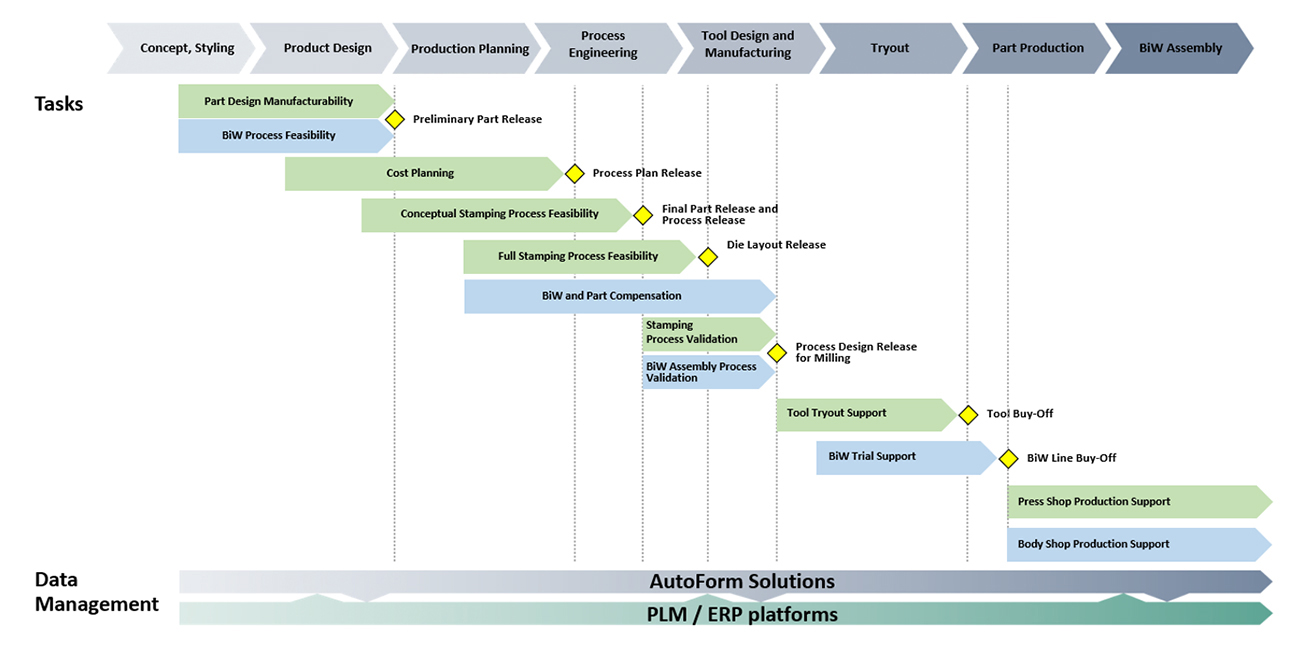
Fig. 7: AutoForm’s Complete Coverage of the BiW Assembly
and Stamping Process Chain
Best-in-class methodology development to educate customers and train users in the optimal and most efficient problem solving methodologies:
These digital twin methodologies are available to AutoForm customers as part of a complete service offering for trainings and technical consulting. Each methodology is using state-of-the-art software functionality and contains deep know-how regarding the workflow and boundary conditions which need to be observed in order to ensure a successful engineering outcome.
The totality of AutoForm’s offering uniquely satisfies the requirements of Industry 4.0 and has the potential for full digitalization of the BiW process chain underscoring AutoForm’s market-leading position. Automotive OEM’s worldwide are leveraging this unique offering and know-how to secure AutoForm’s assistance in their drive to implement state-of-the-art Industry 4.0 strategies in the stamping and BiW assembly process chain.
The totality of AutoForm’s offering uniquely satisfies all requirements of Industry 4.0 and the full digitalization of the BiW process chain.
Modelling Prerequisites to Achieve Highly Accurate “Digital Process Twins”
Just like in the previous section regarding the suitability of software systems, the modelling process and the modelling standards need to satisfy certain prerequisites in order to ensure the success of fully digitalizing the process chain.
The engineering analysis, that is, the “digital twin” or the computer model of the production process developed during process engineering and validation, must be accurate enough to model all important causalities which will have an impact on the actual production process. That is, materials, tribology and lubrication, machine and tool kinematics and forces, tool geometries, and their typical real-life fluctuations which occur in a typical production environment must be considered, modelled and validated.
In this context, the most useful definition of engineering accuracy is the capability of the engineering process to perfectly and fully realize the goal of full digitalization – namely the design and engineering of production processes which run smoothly and which are fully capable of dealing with all parameter fluctuations of the production process which can reasonably be expected. If the software systems and the digital twins are capable of predicting and avoiding all production problems, then the analysis has achieved perfect accuracy.
Simulation Accuracy
If the software systems and the created digital process twins are capable of predicting and avoiding all production problems, then the simulation has achieved perfect accuracy.
AutoForm is providing meaningful and comprehensive guidance to users and customers regarding best model-building practices. The Concept of the AutoForm Accuracy Footprint provides a very practical and easy-to-use guideline to building accurate digital process twins which are fully capable of consistently achieving highly accurate results. This will be presented in more detail in a subsequent chapter.
Since the engineering process of sheet metal parts and BiW assemblies is an iterative process involving frequent reassessments and updates of the engineered production process to reflect updated part geometries, the AutoForm Pareto Principle [[07] Bart], ([08] Bart), [[09] Bart], [[10] Bart], [[11] Bart], [[12] Bart] was developed. To support the model evolution during the engineering analysis phases, this guideline for users prioritizes concrete modelling parameters and specifies the sequence in which certain parameter families need to be ascertained and validated in the engineering process starting with the most and ending with the least influential parameters.
Fig. 8: The AutoForm Pareto Principle Explained
Consistency is also of great concern for the modelling effort – after all, engineers need to achieve consistently good results, not just occasionally or sporadically. AutoForm software is supporting the assurance of modelling consistency, by indicating non-consistent data inside the model, and by providing functionality directly in the AutoForm GUI to ensure model-building consistency throughout the engineering process.
Requirements for the Product Development, Engineering and Creation Process
Assuming that software fully capable of supporting the full digitalization of the BiW process chain is used and that modelling efforts result in highly accurate physics-driven digital process twins, the benefit of the engineering effort can only be realized if the workflow processes are designed to fully realize and implement the results.
The product development, engineering and product creation process needs to be capable of delivering the digital twin analyses to the downstream and upstream stakeholding departments and teams and to drive the decision making processes with meaningful predictions.
After all, the best engineering analysis does not generate any value if the analysis results are not known, not accessible or impossible to interpret by other departments and teams which could have profited from the human intelligence invested in the analysis.
Some examples for illustration:
The most important paradigm regarding the full digitalization of the process is that the digital model must be used as the blueprint for the creation of the physical process. This principle is encapsulated in the paradigm statement “Reality matching simulation”.
Reality Matching Simulation
means that the digital process twins created during process engineering are unconditionally accepted as the master plan and serve as blueprints
for exact replication in the physical world.
This may seem like a very ambitious goal since it is clear that not all secondary and tertiary effects (e.g. ambient temperature and humidity in the production plant) observed in the physical world can be or must be modelled. What this means in practice is that minor discrepancies between the digital twin and the physical representation of the process – the physical twin – are to be expected. The key to success lies in the management of dealing with these effects.
If the major and important physical dependencies are part of the digital twin models, there must be a physical reality that will work. To ensure success, all important process parameters need to be controlled and adjusted in complete alignment with the digital twins being used as blueprints for the physical twins.
Only if the entire workflow pursues the goal of an as-complete-as-possible match of the physical world with the pre-existing virtual world, i.e. if we accept the digital twin as the master plan and if we do our best to exactly replicate the digital twin in the physical world, will we be able to systematically identify and eliminate the remaining small discrepancies between digital and physical twins to achieve perfect matches in the future. This clearly must be the goal of the digitalization and Industry 4.0 efforts.
From this approach, it seems clear that this requires intimate collaboration of all involved departments and teams and that structured upstream feedback from manufacturing to engineering is essential. Of course, it is also obvious that paradigm “Reality matching simulation”, also establishes a very high quality and accuracy expectation on the simulation model, i.e. the digital proces twins. It is just as important that the simulation model and the digital twins can actually be built in the physical world, i.e. the models built must be achievable in the physical world. In order to ensure this, the model building process must follow the model building standards, procedures and guidance provided in the section “Modelling Prerequisites to Achieve Highly Accurate “Digital Process Twins”.”
Chapter 3: Digital Process Twins and the Significance of the Physics-Driven Digital Twins
The Digital Process Twin
Today, many automotive production facilities (OEM or supplier-operated) are tracking the production process using sensors and cameras generating large volumes of measurement and image data. With reference to the Industry 4.0 implementation scale introduced in Fig. 1, these activities represent Level 3 providing full “Visibility” of what is happening in production at the moment. By digitally processing this data, production facilities can, for example, support and streamline their maintenance scheduling and quality management systems.
Furthermore, by using Artificial Intelligence (AI) methodologies this data may further be processed to detect dependencies of input parameters and production process outcomes. In this way – i.e. by identifying the process window and by systematically controlling and adjusting important production process parameters – production line downtimes may be reduced, productivity increased and production scrap avoided. ([13] Schuler Pressen GmbH)
Such AI-based algorithms have the potential to identify systematic relationships between parameter variations and the production outcome and can therefore also generate some predictions achieving Levels 4 and 5 of the Industry 4.0 implementation scale. Many automotive companies engaged in production activities are researching and are actively searching for practical solutions in this field.
In essence, the tracking data is used to create an image of the production process and many companies are trying to use such “digital process twins” to control the production process to stay inside the optimal process window. Since such digital process twins are based on sensor data alone, they will be referred to as “data-driven digital process twins” in the context of this publication.
Since all of the data used to build the process twin are generated during production itself, the above-described use of production-generated data for the solution of production-related issues must be considered an isolated digitalization of one particular phase of the product life cycle. Since in this case, the digital engineering process is disregarded, this process falls short of a comprehensive, full digitalization of the entire product development process and leaves crucial questions unanswered.
As described in Chapter 5: Challenges and Opportunities of this publication, using a comprehensive digitalization approach which includes the digital engineering process generates significant additional savings and improvement potentials which are capable of reaching the ambitious cost and time reduction goals which many OEM’s are striving for.
Advantages of the Data-Driven Digital Process Twin
The first advantage of the data-driven digital process twin relates to its relevance. The sensor-generated production data from the actual production process is always relevant in the sense that the data is directly extracted from the actual process which is to be improved. The gathered data may not be very helpful for solving a specific production problem – because the collected data measurements may not reveal any connections to the production issue in need of correction. In this case, there might be other measurements and tracking systematics better suited to solving the problem. But the data is always relevant since the data originates directly from the object of study. The relevance of the data for different parts and different production processes is undefined and must therefore be evaluated very carefully and reservedly.
The second major advantage of the data-driven digital process twins are that the data generation for the process twins development are in direct control of the production unit itself. This means that no data needs to exchanged to or from other departments (product development, engineering, tool shop, production engineering, etc.) and therefore this approach lends itself to rapid deployment in production.
The Physics-Driven Digital Process Twin
Today, automotive planning and engineering departments perform in-depth process engineering for stampings and BiW assemblies in advance of any tryout and production activities. These engineering activities are driven by simulation-based models and result in detailed digital process models revealing all important causalities and dependencies involving – among other things – the geometry of the production tooling, measurement concepts, jig and fixture geometries and set-ups, raw materials used for production, production machine parameters, and much more. This data is the product of human intelligence and experience of design and engineering staff and – depending on the type of computational analysis used – can answer questions relating to all important causalities impacting the production process.
Furthermore, today’s simulation technology does not only allow the detailed analysis of forming and assembly processes assuming nominal input data but is also capable of predicting production process robustness, process sensitivities and virtually all concerns which are typically part of the production and quality assurance process itself. Computer models based on the modelling of all applicable laws of physics, capable of creating detailed process outcome and process sensitivity predictions, are called “physics-driven digital process twins”.
Physics-Driven Digital Process Twins
Computer models based on the modelling of all applicable laws of physics, capable of creating detailed process outcome and process sensitivity predictions, are called “physics-driven digital process twins”.
It seems obvious that “reverse-engineering” the causalities of the production process using artificial intelligence – instead of using the “human intelligence” which was encapsulated and validated in the digital process model during the process engineering phase – is inefficient and does not leverage the investment in the development and process engineering process.
Advantages of the Physics-Driven Digital Process Twin
It seems obvious that methodologies relying on sensor-generated data from a production process in operation cannot reliably support production start-ups since the process control requires a certain number of measurements in order to start identifying patterns. This means that during the critical production ramp-up phase, the data-driven digital twin is most unreliable. And since process adjustments are frequent during production start-up the sensor data generated during this stage is likely to be of reduced relevance to actual production runs targeting a quasi-steady process state.
Furthermore, the relevance of sensor data generated for similar parts or production processes is questionable and is unlikely to bring substantial improvements for an actual production process ramp-up.
However, physics-driven digital process twins not only have the potential to be used from the very first production ramp-up activities on, but – in fact – should be used to guide the ramp-up in order to directly create the production process which was previously engineered.
The physics-driven digital process twin models and allows the recognition of causal relationships and dependencies which are hard or even impossible to identify using the data-driven digital twins approach. Since the number of process parameters impacting the robustness of a stamping or BiW production process is actually much greater than the process parameters undergoing variations in a typical production environment, it is impossible to identify a non-robust process and to devise counter-strategies using the data-driven digital twins methodology. In other words, it is unlikely that sensor data will suggest strategies how to fix a non-robust process since data-driven digital twin models cannot recognize any causalities, especially involving data which is or cannot be measured.
A practical example for illustration purpose:
During press shop operations, generally there are only a handful of parameters available to the press operator to adjust the stamping production. These parameters include, for example, the lubrication amount (and possibly the lubricatioin pattern on the blank), the blankholder force, and the stroke rate of the press. These are, of course, also parameters which can be measured.
Measurement data of typical production tracking will, however, not be able to identify the problem root cause if the robustness of the production process is compromised by, for example, a non-robust drawbead set-up, or insufficient spotting of the tool since these parameters lie outside of the scope of the measurements. Such causalities can only be identified by taking a comprehensive look at the process modelling the physics of the process with all parameters impacting the production result.
The Challenge of the Physics-Driven Digital Process Twin
While the advantages of physics-driven digital twins are enticing, there is, however, a downside. The benefits of digitalization are substantial, but they require investments and process adjustments.
Unlike for the data-The relevance of the physics-driven process model data for a particular concrete production process is not automatically given. This is – strictly speaking – only the case if the actual production parameters match the parameters used for the process simulation perfectly. For example, if the process validation performed during engineering phase is based on data which is not identical with production data – let’s take, for example, the blank size and material data for a stamping operation – then the relevance of the simulation model for the actual stamping operation may – in fact – be very low. And – given the complexity of BiW/stamping production operations – it is a complex task to establish the full relevance of the engineering data for the actual stamping operations.
What this means is that the use of the physics-driven digital process twins – and the re-use of the engineering know-how encapsulated in the process simulation model – must be ensured by the automotive development process. This is particularly important for the transition from the virtual (data) to the real (physical) world. The development process must ensure the relevance of the digital process twins for the subsequent production operations. The ramifications of this important paradigm will be explored in more detail later on.
Hybrid Digital Process Twins
Since data-driven digital process twins do not use any results of the digital engineering process, it seems plausible that its prediction capability will increase if engineering data, such as tool geometry, and other important process parameters, are included in the data model.
However, this would have the disadvantage of being reliant on engineering data – and would eliminate one advantage of the data-driven digital process twin, as discussed above.
On the other hand, it also seems plausible that a physics-driven digital process twin should generate more accurate and relevant predictions if actual production data is included in the prediction model.
Hence, a combination of both technologies promises advantages. The feasibility of such approaches will have to be explored in the future.
Chapter 4: Building the Physics-Driven Digital Process Twin
Evidence-based decision making using the process approach recommended by independent engineering and standardization organizations
ISO
Professional associations and standardization organizations have defined guidelines to provide guidance to engineers how to assess the quality of their processes and engineering tools – such as , for example, the 7 quality management principles described in ISO 9001-2015: QMP1 “Customer Focus”, QMP2 “Leadership”, QMP3 “Engagement of People”, QMP4 “Process Approach”, QMP5 “Improvement”, QMP6 “Evidence-Based Decision Making”, and QMP7 “Relationship Management”. ([14] International Organization for Standardization, ISO.org)
Engineering work is generally a highly collaborative effort involving engineers and other professionals from different teams and departments. Hence, in line with the quality management principles QMP4 “Process Approach” and QMP6 “Evidence-Based Decision Making” of ISO 9001-2015, engineers need to use software tools which
A very useful guideline to gauge to which degree software tools and physics-driven digital twins support engineering collaboration is the standard ISO/IEC TR 24474:2021 Systems and Software Engineering –Life Cycle Management – Guidelines for Process Description – Guide to the Software Engineering Body of Knowledge (SWEBOK) ([15] IEEE Computer Society).
One important concept outlined in the standard is CONSISTENCY. Consistency in this context concerns the degree to which the computer model used for simulating the manufacturing process (stamping, BiW assembly, etc.) is free of conflicting or incompatible requirements, assertions, constraints, functions, or component descriptions in all knowledge areas. However, there are also other aspects of consistency such as the use of adequate software technology suitable for the analysis objective and the reliability or accuracy of the input data. The ISO-mandated process approach states that consistent results ensuring high prediction capabilities are effective and efficient when activities are understood and managed as comprehensively interrelated processes throughout all organizations and systems.
Generally, of course, the achievable prediction accuracy of the algorithms in use should be commensurate with the reliability of the input data and modelling accuracy. An example of the violation of this type of consistency would be the use of rough estimation algorithms on highly mature and accurate input data – and vice versa.
The direct consequences of the ISO 9001 requirement for software tools are clear:
ASME
The American Society of Mechanical Engineers (ASME) Standards Committee on Verification and Validation in Computational Solid Mechanics have created standards for assessing the correctness and credibility of modeling and simulation in computational solid mechanics. ([16] The American Society of Mechanical Engineers) The initial guideline approved and published in 2007 is being considered as the basis of ISO processes in model-based systems engineering to enhance innovation productivity. This is identical with the concept of physics-driven digital twins.
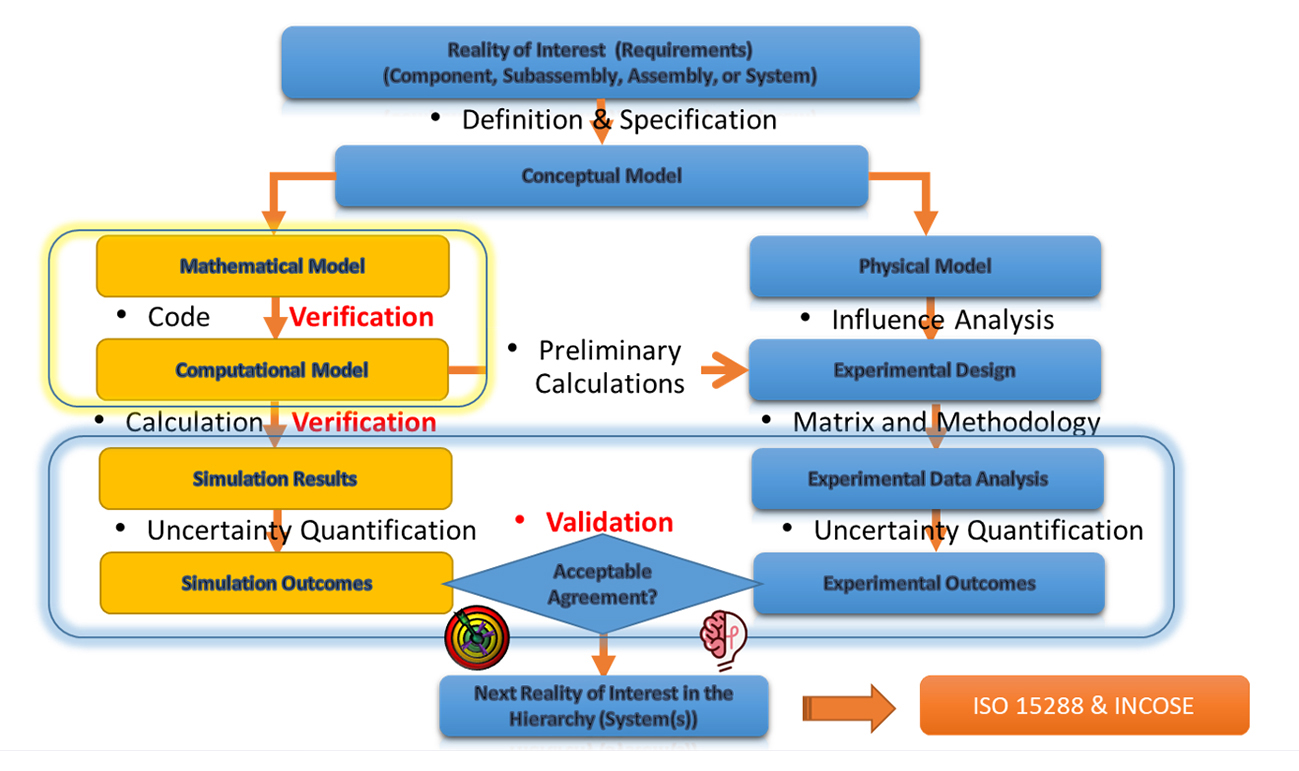
Fig. 9: Flow Chart of Verification & Validation Activities and Outcomes
Recalling the section on The Three Pillars of Digitalization in the Context of Manufacturing Simulation, software verification preceding model validation should also include an uncertainty quantification in alignment with the ASME guideline of model credibility.
Typically, the physics-driven digital process twins are create before the physical twins and the validation process should therefore be that physical twins match the physics-driven digital twin before checking validation. In validation, it is clear that the uncertainty quantification is necessary to statistically approve the match rate between physics-driven digital twins and physical twins. The key message of these verification and validation activities are:
The AutoForm Accuracy Footprint
The AutoForm Accuracy Footprint methodology is an easy-to-use methodology to ensure consistently high modelling and result quality of manufacturing process simulations. It represents a tried-and-true, practically proven systematic approach to comply with the requirements mentioned in the previous chapters for the creation of high-quality physics-driven digital process twins.
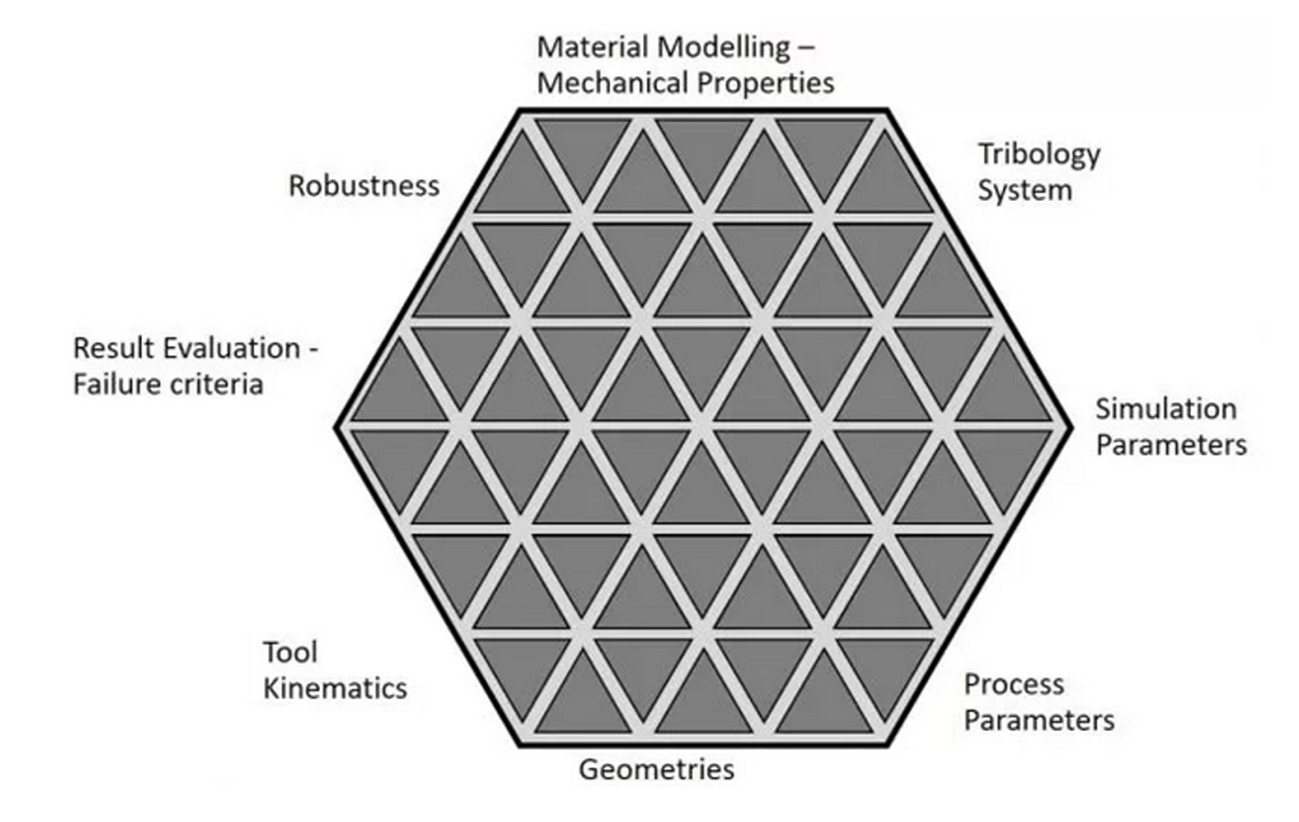
Fig. 11: Visualization of the AutoForm Accuracy Footprint Concept
These 8 aspects of computer model /digital process twins creation need to be kept in mind to achieve the engineering objective and to build accurate computer models and digital process twins. The practical implications of this methodology are manifold. Let’s consider a few consequences of this modelling methodology:
The AutoForm Accuracy Footprint
helps create consistent, valid and accurate digital twins modelling stamping and BiW production processes.
Process design defining process aspects such as draw bead design and die surface layout can be started with the material specs and part geometry provided as initial conditions from part or product design. During subsequent process feasibility studies, the major job of process engineers is to update the geometries with tool kinematics and to further develop or update new process aspects to achieve the relevant part quality for future production based on the evaluation standards.
Importantly, these updates need to satisfy the engineering goal of robustness with realistic inputs of the variations of production conditions (e.g. tribology, tool clearances, parameter variations, etc.) and proper evaluations. These updates to the computer model are gradually and continuously taking place during the whole engineering process maturing the process design gradually to production level.
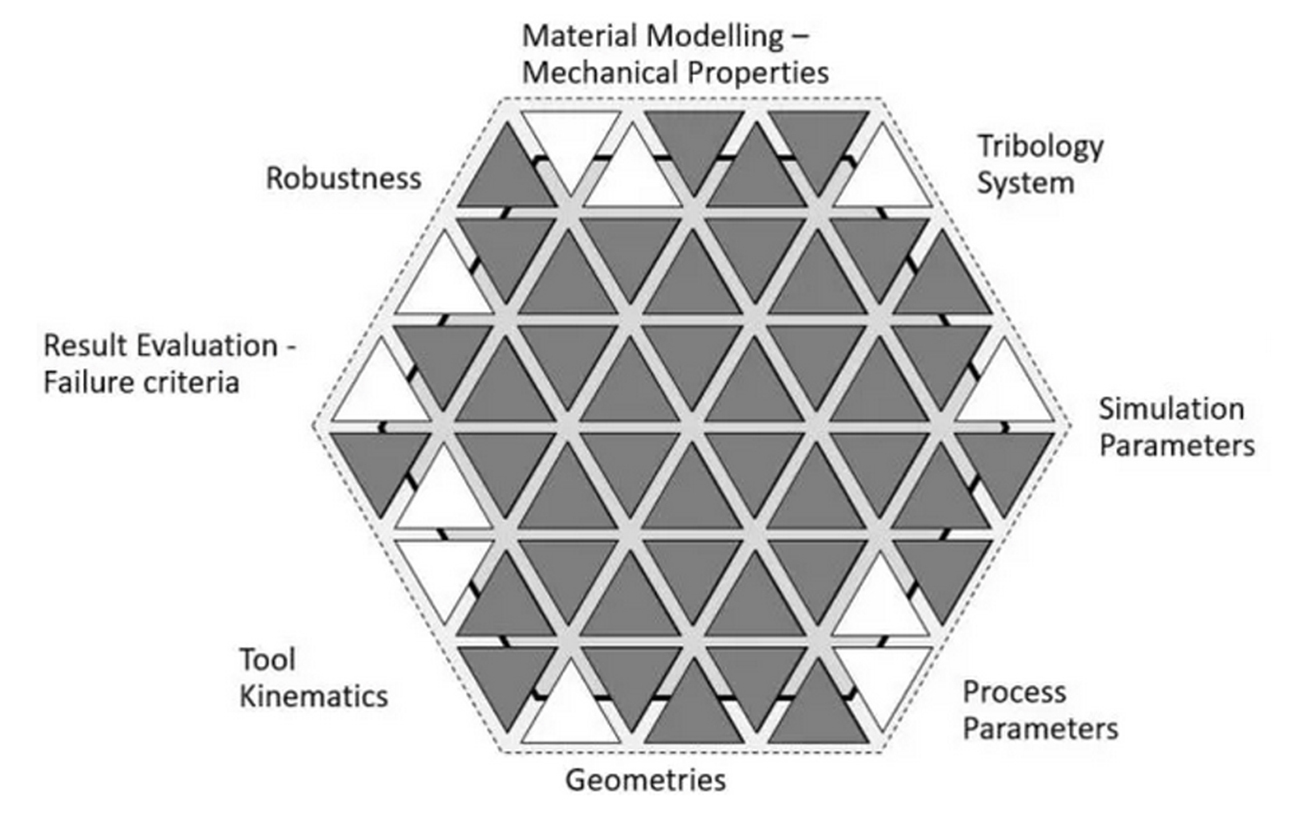
Fig. 12: Visualization of a Partial Accuracy Footprint
In practice, users of sheet metal simulation software are often faced with seemingly non-matching results from stamping trials with their simulations. The AutoForm Accuracy Footprint can serve to systematically drill down to the root cause(s) of the result discrepancy. If one or several parameters do not match – in the digital and the physical process representations – then you have a reduced accuracy footprint. Consequently, you can expect deviations between simulation results and experimental results. Differences in input on either side is why the results don’t match. The root cause analysis often takes on similarities to detective work digging deeper into the intricacies of a production process until the “culprit”, i.e. the mismatched process aspect, is found. ([18] SchaefflerDaniel)
Process Robustness/Capability Assessment – A Key Requirement for Accuracy and Industry 4.0
One important and indispensable aspect of the AutoForm Accuracy Footprint methodology outlined above is the evaluation and quantification of the process capability of the designed manufacturing process in line with ISO quality management principles – especially the ISO process approach and evidence-based decision making – as well as with the ASME guide line for the prediction accuracy of physics-driven digital twins. The reason for the importance of this aspect is two-fold and deserves to be discussed in some more detail.
Firstly, “Accuracy” is a concept which literally only makes sense in the context of multiple data sets and stochastic analysis. By comparing two different data points with each other, an assessment of any kind of accuracy is simply meaningless. The validation and verification concepts developped by ASME reflect this fact.
To illustrate this simple, but often-ignored fact, let’s consider the following realistic scenario: a measurement result (let’s say, the part springback measurement at a specific point on a stamped panel) is compared with the corresponding springback result of a forming simulation. The graphical representation of the results are shown in the following figure.
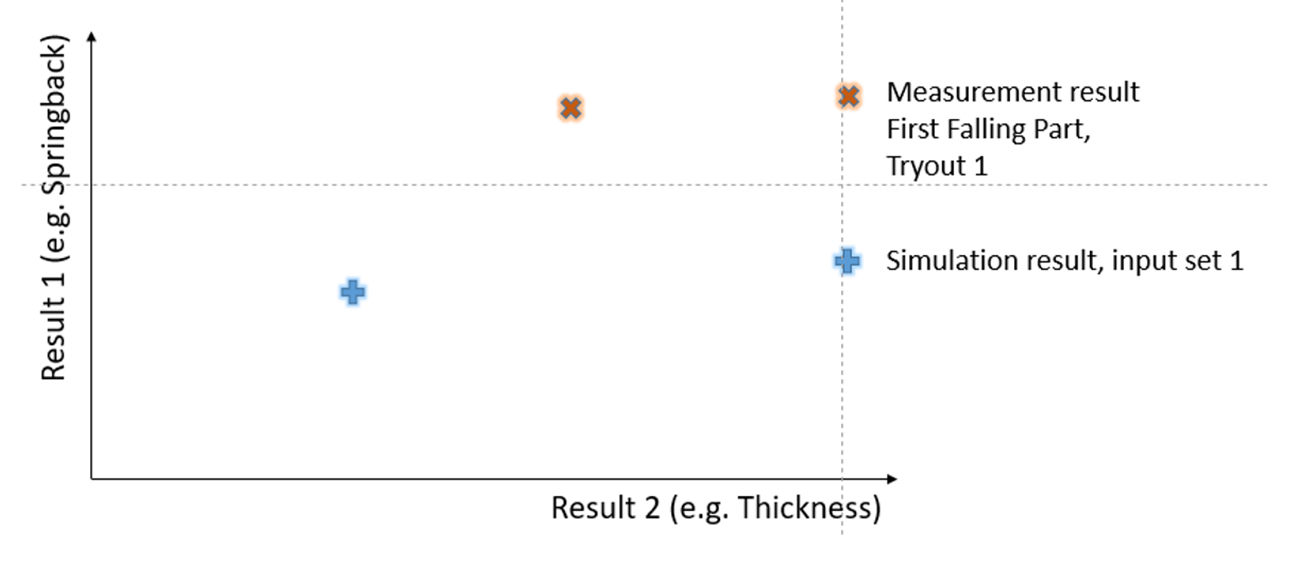
Ffig. 13: Comparison of Measurement and Simulation Result - First Try
Clearly, the graphic shows a discrepancy between the result of the measurement and the simulation. In light of the discrepancy, often times the question arises whether this is a good result or a bad result – is it accurate or not? One might consider the “percentage” of the deviation to estimate the accuracy – or, better, search for the reasons and root cause for the discrepancy.
To investigate the question of accuracy a bit further, let’s assume, a second trial is conducted and the simulation result is modified to reflect (for example) slightly different material characteristics of the blank. The analysis is repeated and the following chart is produced:
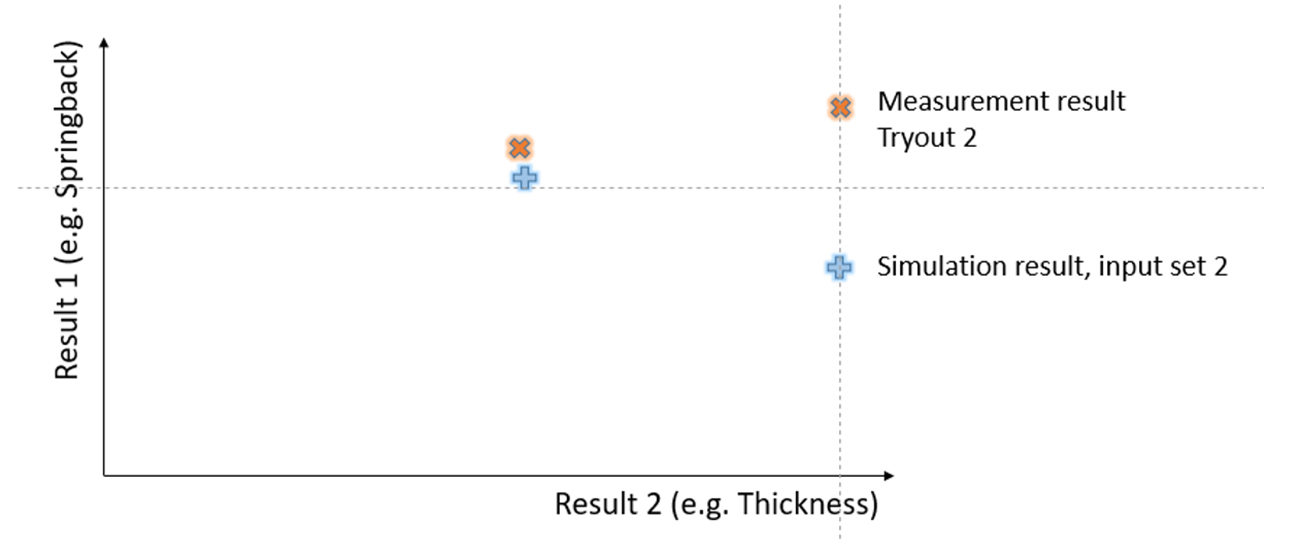
Fig. 14: Comparison of Measurement and Simulation Result – Second Try
This looks much better, doesn’t it? But what does it mean? Is the second simulation “more” accurate – in the sense of “closer to the truth” than the first simulation?
To answer this question, let’s assume, the springback is tracked during production for some time. And after running a robustness analysis evaluating typical production parameter fluctuations, we arrive at the following summary:
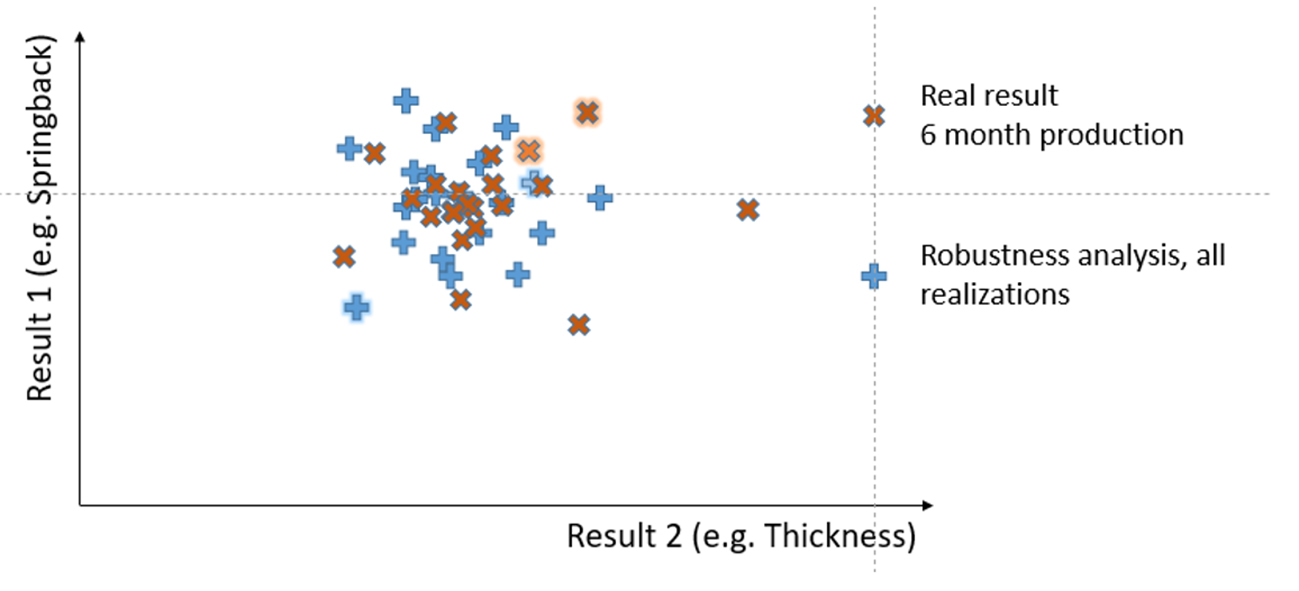
Fig. 15: Comparison of Measurement and Simulation Result Data Sets
Now we recognize that the data points measured earlier are part of a larger cluster – this, of course, is always the case in the real world of automotive production. Furthermore we see that the measurements show some scatter (this is also always the case) and that the simulation scatter covers approximately the same area as the actual measurement scatter.
We realize that now we can reach a meaningful assessment of the accuracy – or the match of the two data sets. And we also realize that a comparison of individual data points for a production process (physics-driven digital or physical) has almost no meaning – it certainly cannot assess the accuracy of a simulation or a manufacturing process.
So – the concept of accuracy (and its counterpart, the result precision) – is inseparably connected with the concept of stochastics and data sets. That is, multiple data points or data clouds. Hence, the assessment of simulation accuracy requires a stochastic analysis of the manufacturing process – in other words, without robustness analysis and statistically-quantified analysis, no accuracy assessment and also no evidence-based decision making in engineering phases are possible.
Fig. 16: Understanding What Accuracy Means [[19] Intaek]
The second compelling reason for including robustness assessment of the manufacturing process in the AutoForm Accuracy Footprint methodology, is the goal of the process engineering activities itself. The goal of the process engineering is to create – beginning with the part geometry and material specification – a production process satisfying all functional, quality, cost- and time-related targets. In particular, the designed process needs to ensure a robust, smooth production process with maximal productivity (i.e. a minimum number of unplanned disruptions, the lowest production scrap rate, etc.).
This can only be achieved if the actual production robustness – on the basis of the designed or intended manufacturing process – can be simulated, quantified and hence optimized. Running one or several discrete simulations using discrete input data sets falls short of this goal and therefore does not support the digitalization strategy.
The AutoForm Pareto Principle
Aligned with the ISO process approach to quality management, the AutoForm Accuracy Footprint methodology outlined above focusses on the “bases that need to be covered” by the engineering process in order to ensure high-quality outcomes. In practice, this principle needs to be augmented in order to take the iterative and evolutionary product development and process engineering process into account.
What this practically means is that in the beginning we start with a clean slate or very little information – geometry, some tolerances and material specs. And possibly a reference process which can be used as a process blueprint. During the development and engineering process more and more information becomes available, more product and process related decisions are made – and the part geometry as well as the production process design gradually mature and become more refined.
To respect and integrate this maturation process into the AutoForm Accuracy Footprint methodology, the AutoForm Pareto Principle was created. Hence, the AutoForm Pareto Principle mirrors the engineering process beginning with a rough definition and refining the initial concept successively to its final completion.
The Pareto principle states that 80% of outcomes are the consequence of only 20% of causes. This principle was developed in the context of quality control and quality improvement and named after the Italian economist Vilfredo Pareto. Mathematically the 80/20 rule is described by a power law distribution. Many natural phenomena have shown to adhere to this law.
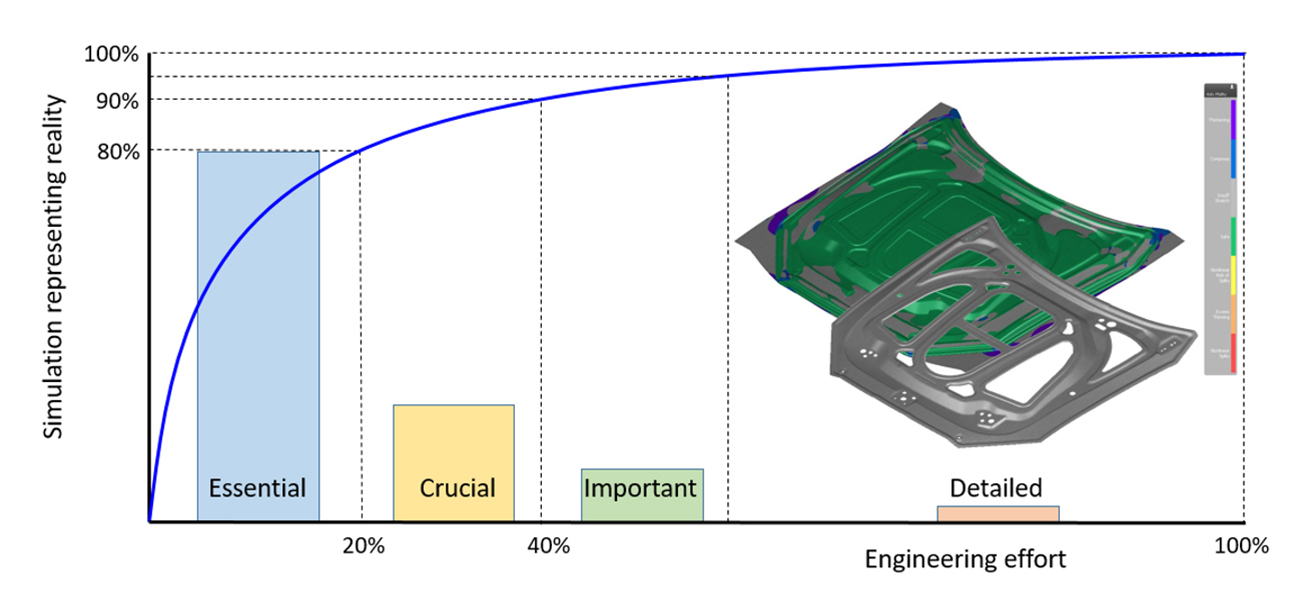
Fig. 17: Simulation Input Parameters and their Significance for the Simulation Result
The Pareto principle in engineering can be used to simplify the creation of complex stamping and BiW digital process twins. Following the ISO process approach the Pareto principle reduces the complexity and integrates the whole process to allow easier and quicker engineering processes. As shown in Figure 17, the engineering model-building process can be discretized into 4 steps:
The AutoForm Pareto Principle was designed – among other uses – to systematically and consistently achieve the highest possible simulation accuracy. To start, the essential input parameters need to be identified – i.e. those which influence simulation results most significantly. Knowing and validating these parameters, we create the basis for a simulation to represent 80% of the future shop floor reality.
The AutoForm Pareto Principle
was designed to systematically and consistently achieve the highest possible simulation accuracy.
The essential parameters for a stamping simulation setup involve:
Having secured these parameters, the process may be refined further as additional details about the manufacturing process become available. The other parameter classes are similarly and concretely defined and classified.
In this way, the AutoForm Pareto Principle does not only provide guidance on the impact of different parameters on the simulation result, but it also suggests an engineering-based model building process working from the more significant to the less significant input parameters. In this way, it helps to systematically build a consistent and accurate simulation model.
The maturation of the process definition goes hand-in-hand with the maturing simulation model. The more simulation-supported decisions are made, the more the process matures and the simulation increasingly represents the future, not-yet-existing reality and is converging towards true accuracy. The ultimate level of accuracy is achieved when the production result matches the simulation result.
The implication for the process chain is that the physical process has to be a representation of the engineered virtual process, and this is exactly why we put all effort in engineering in order to achieve a very smooth tool tryout and, later on, a very smooth production of the part.
Connection to and Significance for Industry 4.0
The connection of stochastic engineering analysis to Industry 4.0 and the digitalization of the process chain is the creation of production-relevant data during the engineering process. Smart engineering paves the way for smart production achieving the highest implementation level on the Industry 4.0 scale: the autonomous production (see Figure 1). The modelling data, i.e. the physics-driven digital process twins encapsulate all the human intelligence that went into the engineering of the manufacturing process.
Provided that the physical state of the process (tool geometry, sheet metal material used, press kinematics, order of weld points in the BiW assembly, etc.) is a true image of the digital twins, the engineering data can directly, i.e. without modification or enhancements, be used for ramp-up and production control and guidance.
The use of stochastic simulation data to drive stamping press controls and BiW assembly equipment is one of the final connections to establish for completion of the full digitalization. First projects indicate that is technically feasible. It will pave the way to far more productive press shop and production operations in the future.
Fig. 18: Simulation-Based Inline Press Control [[20] Andreas]
Chapter 5: Challenges and Opportunities
Challenges
In a recent publication, Dr. Corrie Block, a Forbes Council Member, pointed out that the chances of failure of digital transformation (DX) projects are dauntingly high. Most large consulting companies involved in DX projects estimate the failure chances to be between in the range of 70 to 95% percent.
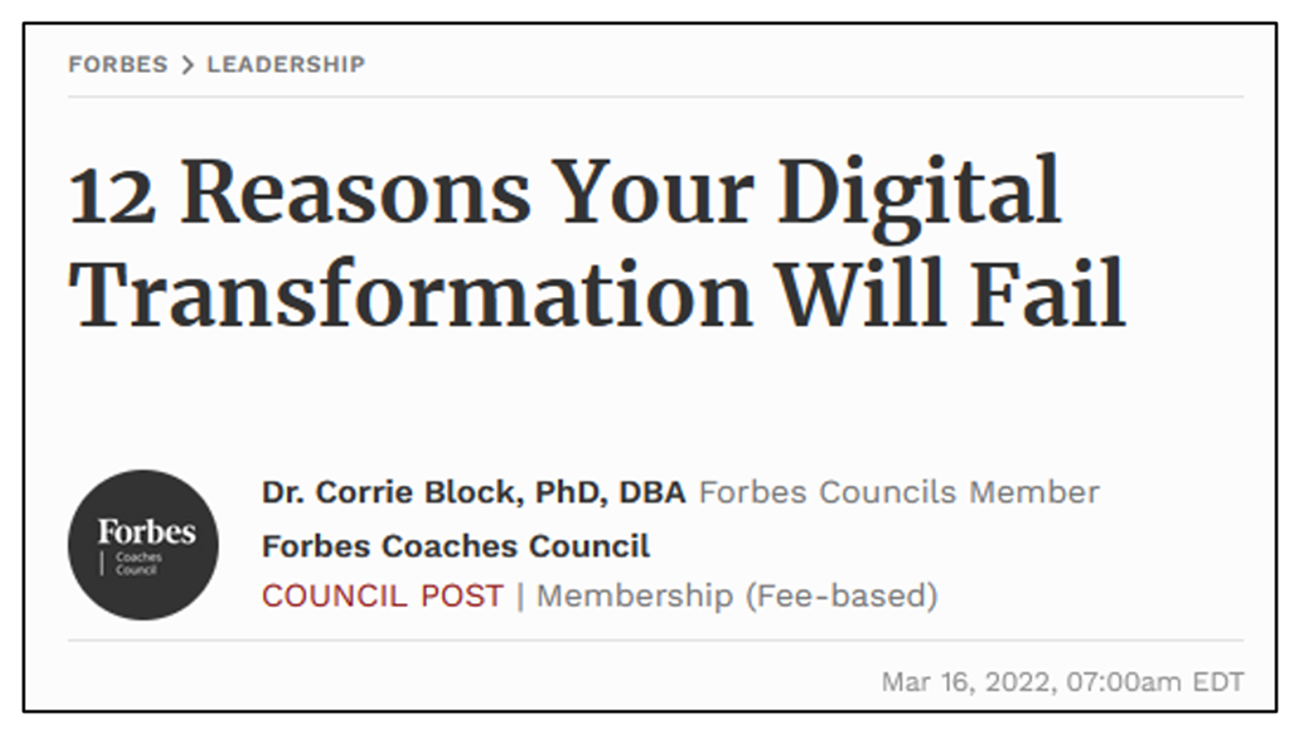
Fig. 19: Publication from Dr. Corrie Block
Dr. Block puts two reasons on the top of his list of reasons for failure:
These experiences clearly point to the necessity that – above all – companies need to work hard to quantify the savings in terms of time and money of their intended DX project before the project is rolled out. While this seems obvious, it appears that not in all cases this due diligence is performed.
Value Quantification is Key
Companies intending to deploy digital transformation projects need to have a very clear picture on the quantifiable benefits and the financial outcomes of the transformation.
Potential Savings
So, in light of the previous section of this paper, let’s take a close look at the possible benefits of the digitalization of the BiW assembly and stamping process chain.
How can the implementation of digital process twins in the stamping and BiW assembly value chain contribute to the efficiency gains discussed in the introduction of this paper?
To answer that question, it is necessary to consider the key value drivers of full digitalization, namely lead time, costs, and quality (see Figure 20). In terms of lead time, digitalization can reduce the time needed for tryout and production ramp-up. In terms of costs, digitalization can reduce production, material, tryout and tooling costs.
Finally, in terms of quality, digitalization can improve part and process feasibility, dimensional accuracy, and surface quality. Together, these drivers make up the value proposition that full digitalization can deliver to the automotive industry.
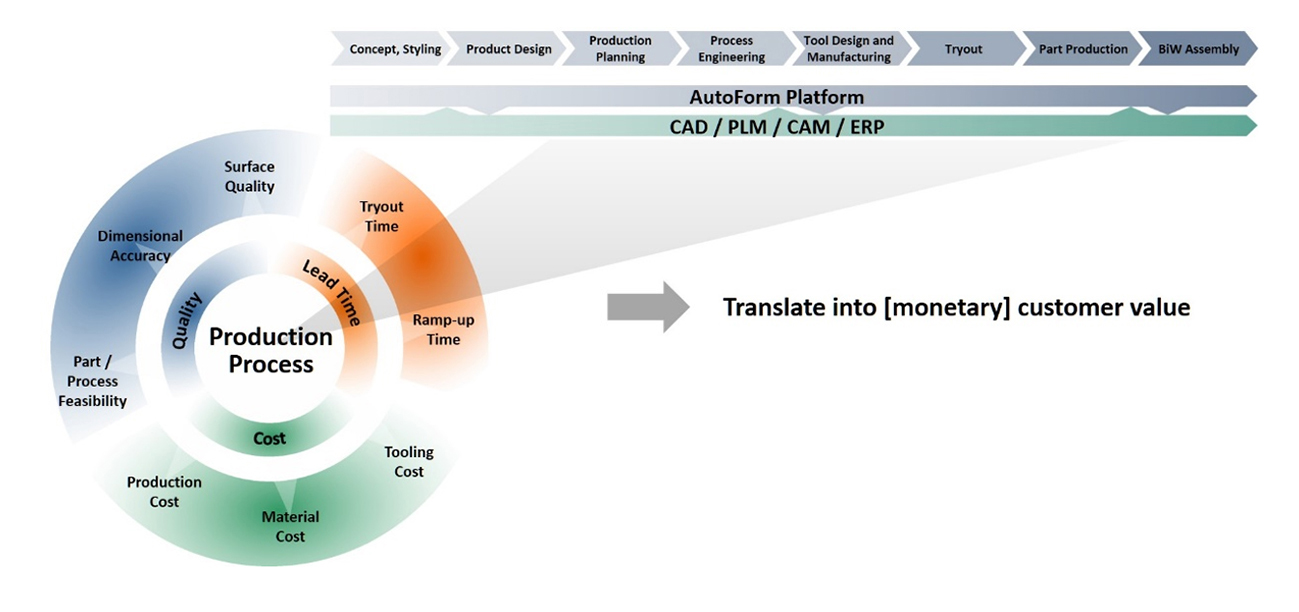
Fig. 20: Value Drivers of the Digital Twin Process
The value drivers will translate into quantified tangible savings, in all stakeholding departments and areas involved in the process from product inception to mass production. The list of beneficiaries and stakeholders of a full process chain digitalization is impressive: product development, GD&T teams, feasibility engineering, cost and value engineering, process design and engineering, production engineering, tool design and tool tryout activities, home line tryout activities, press shop ramp-up, press shop operations and quality control and management, BiW process engineering, BiW pilot factory trials, BiW ramp-up and production as well as quality assurance and management.
As indicated in Figure 4 automotive OEM’s are aiming at significant cost savings. But they also are looking at shortening the time line for new vehicle development.
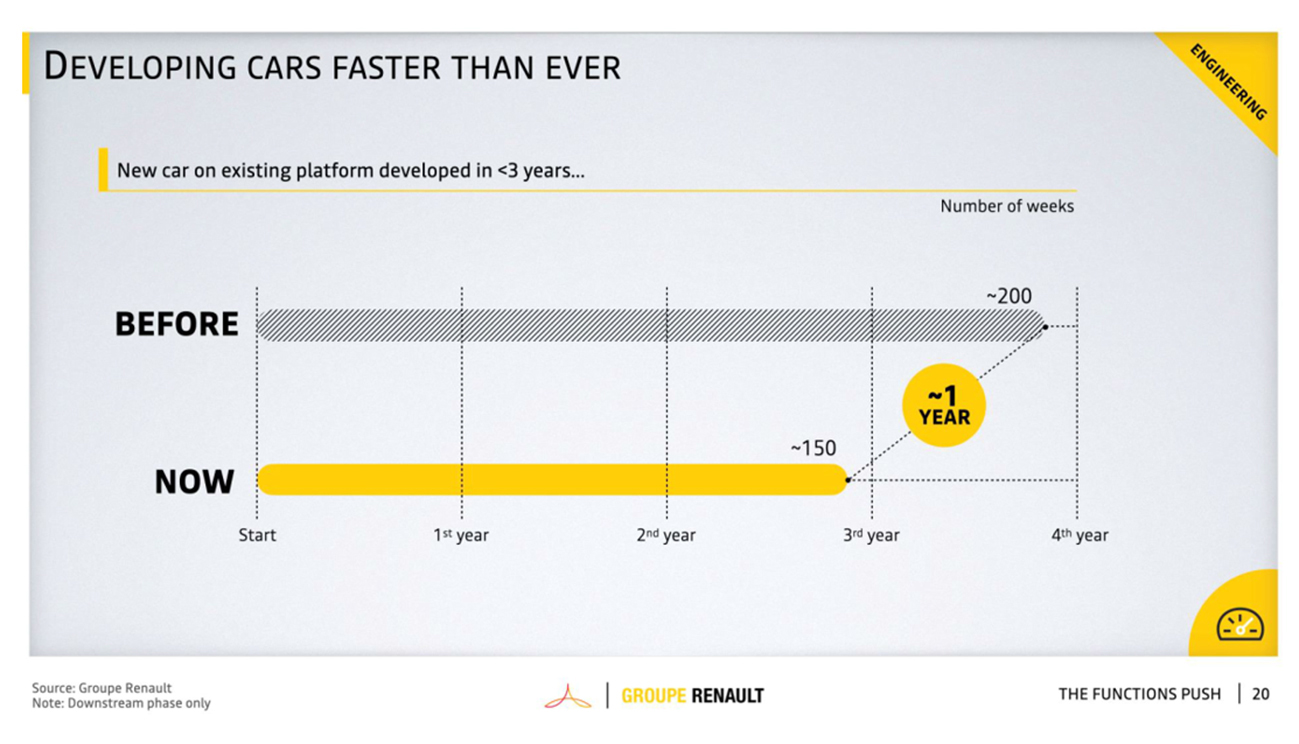
Fig. 21: Time Reduction Goal of the Renaulution Project from Renault (Jan. 2021) [[21] GROUP RENAULT]
On the cost side, full digitalization and the physics-driven digital process twins have the potential to significantly reduce capital (CAPEX) as well as operating expenditures (OPEX). Capital expenditures regard the up-front investment of vehicle-specific production tooling such as the cost of stamping die sets, the cost for the BiW sub-assembly and assembly line equipment and the cost for retooling final assembly lines. In the context of the BiW and stamping process chain, the major CAPEX position representing the “low-hanging” fruit for cost reduction is clearly the tool cost.
Still today – even after industry-wide adoption of forming simulation technology – tryout and quality loops in tool shop and home line tryout account for approx. 15 to 40% of the total cost of a stamping tool set. Many long-lead time parts – especially made from advanced sheet materials – experience more than 6 iteration loops, with some reaching more than 10. The major reason for that is part springback. However, even after the tool is modified to produce the stamping within all dimensional specifications, additional change requests come in from the BiW department to ensure assemblability.
The physics-driven process twins – and adoption of a fully digitalized process chain – these costs can be reduced by more that half. The goal must clearly be to eliminate all physical tryout activities and reduce the tool tryout activities to a mere validation of the digitally engineered process. As indicated earlier in this paper, the achievable reductions in tool cost alone may easily exceed 10 Mio. € per vehicle project.
Elimination of all physical tryout activities
and the reduction of the tool tryout activities to a mere replication and validation of the digitally engineered process must clearly be the goal.
On the OPEX side, savings of similar magnitude are achievable by systematically optimizing material utilization and optimizing production process robustness which will lead to minimization of unplanned press line down-times, reduction of the production scrap rate, speed-up of production ramp-ups and an increase in the overall average stroke rate.
To reduce the vehicle development time by a full year (see Figure 21 above), means that all stages of the process chain need to deliver the correct processes and parts in the physical world in the first try. There will no longer be any time for trial-and-error activities in the physical world. And problem parts involving more than 10 tool shop iteration loops must reliably be avoided, since such occurences lead to milestone overruns – and possibly jeopardize SOP deadlines.
Lead time savings are achievable through the systematic reduction of tryout and quality loops in tool shop and BiW pilot factory. Through the creation of a digital process twin involving the BiW process as well as the stamping processes, and using an integrated BiW and stamping process chain, mature stamped parts satisfying dimensional and quality specs will become available much earlier than in the past.
Savings of more than 6 months lead time in the creation of the BiW are realistic due to digitalization and use of digital twin methodology. Many automotive OEM’s have recognized this potential and cite increased digitalization efforts as one of the key contributors to the achievement of lead time savings goals.
Large Quantifiable Savings
Digitalizing the stamping and BiW assembly processes will generate large savings in CAPEX, OPEX and lead time.
These savings can be quantified by AutoForm for each project partner using a detailed analysis rooted in a deep understanding of the “as-is” and the “to-be” processes..
Technology and Methodology
Getting back to the implementation of full digitalization strategies in the product development, engineering and production process chain, in Chapter 2: Key Elements and Paradigms for Digitalization of the Stamping and BiW Assembly Process Chains we have already outlined that the model building process and the workflow definition, including milestone and stakeholder definition, are important areas of concern for full digitalization.
Of course, software functionality is important – and has been, in many ways, an innovation driver of modifications in the automotive BiW process chain. However, it seems obvious that just by purchasing more software licenses, the financial and time savings goals will not automatically be achieved. The information and insights generated by meaningful engineering analysis has to be tied into the decision making processes in the process chain in order to generate benefit.
So, the success of the digitalization effort will largely be determined by how well the application of suitable software is tied into a new, digital process chain answering the important questions and supporting the important decisions with reliable and timely digital data. Let’s take a look at some practical examples to illustrate what is meant by that.
These examples illustrate two important conclusions:
Today’s vehicle development timelines often do not grant enough time to the engineering teams to identify and eliminate all downstream process issues. However, everyone knows that the elimination of forming and assembly issues “in the hardware” is far more costly and time-consuming than doing this using digital process twins. So – despite spending more time in the engineering stage to “do things right” – the overall timeline can be reduced significantly by shortening the downstream time allotments.
Technology and Methodology
Getting back to the implementation of full digitalization strategies in the product development, engineering and production process chain, in Chapter 2: Key Elements and Paradigms for Digitalization of the Stamping and BiW Assembly Process Chains we have already outlined that the model building process and the workflow definition, including milestone and stakeholder definition, are important areas of concern for full digitalization.
Of course, software functionality is important – and has been, in many ways, an innovation driver of modifications in the automotive BiW process chain. However, it seems obvious that just by purchasing more software licenses, the financial and time savings goals will not automatically be achieved. The information and insights generated by meaningful engineering analysis has to be tied into the decision making processes in the process chain in order to generate benefit.
So, the success of the digitalization effort will largely be determined by how well the application of suitable software is tied into a new, digital process chain answering the important questions and supporting the important decisions with reliable and timely digital data.
A practical example for illustration purpose:
These examples illustrate two important conclusions:
Today’s vehicle development timelines often do not grant enough time to the engineering teams to identify and eliminate all downstream process issues. However, everyone knows that the elimination of forming and assembly issues “in the hardware” is far more costly and time-consuming than doing this using digital process twins. So – despite spending more time in the engineering stage to “do things right” – the overall timeline can be reduced significantly by shortening the downstream time allotments.
DX Savings
are not generated by technology or software, but rather by
deploying new digital twin methodologies and
changing existing workflows.
AutoForm has developed a set of application or digital twin methodologies which are crucial for automotive companies to accelerate their workflows and to generate significant time and cost savings. These digital twin methodologies cover the entire span of the process and involve all stakeholding departments and teams:
Each of these methodologies involve a more or less intricate engineering workflow to achieve the desired engineering and savings outcomes. Each has their own requirements for model building as well as for analysis and result evaluation details. All are fully and optimally supported by custom-tailored AutoForm software and functionalities. And all of the outcomes of these methodologies are fully and completely consistent with each other – i.e. there are no translation or consistency issues when using AutoForm software sequentially to move a vehicle project through the engineering workflow from start to finish using several or all of these methodologies sequentially.
Each AutoForm digital twin methodology– when executed correctly – generates tangible, quantifiable savings which can be used to create digitalization business cases.
Each AutoForm digital twin methodology
– when executed correctly –
generates tangible, quantifiable savings and can be used to create a business case for digitalization.
As an example, consider the methodology “Prediction of Stamping Production Robustness” described above. If this methodology – integrated with the preceeding methodologies to generate high-quality die faces and running full cycle simulations – is executed properly, it may lead to the following savings in operation expenses during stamping production:
These savings can be quantified (i.e. measured in weeks or currency) using industry-specific benchmark data – or customer data – to build a business case for the digitalization of the process chain involving all methodologies. However, these savings are not generated “automatically” but rather only if the collaboration between the teams and departments follow a process capable of using software results to make timely and accurate decisions. To defined and engineer this new “to-be” process requires an intimate knowledge of these digital twin methodologies outlined above – and a practical and realistic view of how to implement changes in a concrete organization starting with the current “as-is” process.
Putting it all together
In the previous parts of this publication, we have tried to bring focus and meaning into the general, all-encompassing topic of Industry 4.0 specifically for the BiW process chain. We tried to make the topic more transparent, more tangible by giving some very concrete examples for the kind of issues which need to be tackled on the path to full digitalization.
So – at least one important question remains: where to start and what to do first?
It may have become clear that those companies who are serious about their digitalization efforts and who are serious about achieving the tremendous benefits on the horizon will have to understand this process as a deeply invasive transformation process to change the way they have done business in the past. A full process chain digitalization will involve all departments and all operative levels in product design, engineering, tool shop, press shop and BiW production.
Hence, the mandate and the guidance to engage in such projects lies clearly in the hands of executive management.
Furthermore – as in any change management process – a course charted into the future will need to begin with a clear localization of the current position and the intended goal. We need to know where we are and where we want to go in order to chart a course.
This clearly necessitates a project approach which will start with the identification and description of the actual, current “as-is” situation.
After the determination of the current location on the digitalization map, the “to-be” process may be described and defined.
The “to-be” process can only be described leveraging deep knowledge of the possibilities in the process chain with today’s existing engineering tools.
However, it is important to remember that technology alone – i.e. software functionality – does not generate any value be itself. Rather, mastering and implementing proven digital twin methodologies based on and utilizing state-of-the-art digital technologies will be the key to success. In particular, mastery of the following three methodologies will determine a large portion of the benefits and success on the road to full digitalization of the stamping and BiW assembly process chain:
Mastery of these three methodologies
will determine a large portion of the benefits and success on the road to full digitalization of the stamping and BiW assembly process chain.
.
These workflows (“digital twin methodologies”) are fully supported by AutoForm software and application know-how. The AutoForm product portfolio today can be used to build and share accurate physics-driven digital process twins – including all aspects mentioned and discussed in this publication. There is no need to wait any longer for solutions to become available.
Chapter 6: Starting the Journey
Where to Start
Simulation technology, that is, software technology to build reliable physics-based digital process twins for the stamping and BiW assembly process chain, has developped dynamically during the past years. Advances have been made in all aspects – resolution detail of the digital models, modelling capabilities regarding forming and tooling technologies, model size and solution speed, process chain coverage, as well as the availability of prediction capabilities using stochastic analysis approaches – all these digital technologies have matured to become important and reliable tools for solving real-life problems.
Leveraging this technology, many companies have made great advances to digitalize part of their process chain by using the digital twin methodologies described in Chapter 5: Challenges and Opportunities – such as robust springback compensation – to reduce their tooling costs and to accelerate their vehicle development and industrialization cycles.
However, robust springback compensation is just one of many methodologies developped by AutoForm. Hence, very significant opportunities open up to those companies who take careful stock of the capabilities of physics-based digital twin technology today and who are willing to assess the potential this technology presents for their advancement.
What Needs to Be Done?
As described in Chapter 2: Key Elements and Paradigms for Digitalization of the Stamping and BiW Assembly Process Chains of this paper, an implementation of best-in-class Industry 4.0 practices in the stamping and BiW process chain needs to rest on three pillars:
In order to increase digitalization and Industry 4.0 maturity, a holistic approach needs to be taken considering all three aspects.
A logical starting point is an in-depth analysis of the current process specifications, including time lines, milestones and deliverables and an assessment whether the current process utilizes all state-of-the-art software capabilities and best-in-class processes.
The typical scenario with regards to digitalization efforts in the stamping and BiW process chain shows that the process chain of many automotive companies is characterized by disconnected digitalization “islands”. By this we mean that some teams and departments involved in the process chain are already using advanced digital tools and technologies very intensively. For example, stamping and process engineering teams have already a long history of using various simulation tools and functionalities for process definition and validation.
However, on the other hand, there are other teams and departments which do not or cannot make use of the results of such analyses – reducing the overall problem-solving and savings potential. And, there are other teams, such as the BiW pilot factory who often have very limited digital process validation capabilitites and who can start their work only after receiving physical parts from tool tryout.
The Project Approach
As was pointed out in the discussion of the digital twin methodologies , benefit is generated by adopting and implementing the digital twin methodologies decribed in the previous chapters of this paper. These digital twin methodologies reflect best-in-class practices to digitalize the product development process.
Hence, the key to success lies in implementing digital twin methodologies in the engineering process and to re-engineer the product development process in order to make optimal use of the reliable information generated by physics-based digital twins.
This journey does not need to start from ground zero – all automotive companies are using process simulation software already today building computer process models to streamline their planning, engineering and production operations. These highly digitalized process portions need to be expanded and connected in order to build a fully integrated digital process chain making sure all stakeholders are connected to the digital process model and have access to all relevant data to perform their tasks.
A Global Perspective
AutoForm is a globally acting company and possesses a truly global view of the automotive stamping and BiW assembly process landscape. AutoForm is in contact with over 1.000 customer companies worldwide and is deeply familiar with the challenges customers and users face day-to-day worldwide. AutoForm staff has acquired deep process knowledge to help customers master these challenges. This know-how is guiding software development expanding the scope of application of AutoForm software to help customers address real-life issues for over 25 years.
In addition to the global presence and deep process knowledge AutoForm brings a unique set of products, experienced staff and consulting skills to the table. This makes AutoForm your ideal partner to start the digitalization transformation – or to move forward with digitalization efforts – in the BiW, stamping, tooling and press shop operations.
AutoForm is your ideal partner
to start the digitalization transformation – or to move forward with digitalization efforts – in the BiW, stamping,
tooling and press shop operations.
.
If you want to know more about how:
please call your local AutoForm office or visit our webpage AutoForm.com.
.
All content in Digitalization Lab is copyright to AutoForm Group and may not be distributed. © AutoForm – all rights reserved.
List of References
[01] Günther Schuh, Reiner Anderl, Jürgen Gausemeier, Michael ten Hompel, Wolfgang Wahlster. "Industry 4.0 Maturity Index." 2017. https://www.fir.rwth-aachen.de/en/research/research-work-at-fir/detail/i40-maturity-index/. https://www.acatech.de/wp-content/uploads/2018/03/acatech_STUDIE_Maturity_Index_eng_WEB.pdf.
[02] Wiebenga, Jan Harmen. „Untapping the Value Potential of Digital Transformation: The Digital Process Model as Enabler.“ February. 2021. https://formingworld.com/. https://formingworld.com/digital-process-twin-as-enabler/.
[03] Ruggiero, Gianfranco. „Another Link Forged for the Digital Process Chain: AutoForm-TryoutAssistant.“ December. 2017. https://formingworld.com/. https://formingworld.com/tryout-assistant/.
[04] McClanahan, Todd. "See the Video: AutoForm Assembly Launch: Putting the Puzzle Pieces Togather." July. 2021. https://formingworld.com/. https://formingworld.com/autoform-assembly-launch-2021/.
[05] Wiebenga, Jan Harmen. „Full Digitalization of the Stamping Body-in-White Assembly Process Chain (Part 1).“ February. 2021. https://formingworld.com/. https://formingworld.com/automotive-industry-trends/.
[06] Chertes, Dorin, Matrite Dacia. „Adopting the Right Frame of Mind for Successful Simulation: Renault Group Team Leader Oversees 70% Reduction of Man Hours.“ January. 2021. https://formingworld.com/. https://formingworld.com/renault-group-man-hours/.
[07] Carleer, Bart. „A New Way Forward: Introducing the AutoForm Pareto Principle in Forming Simulation.“ August. 2021. https://formingworld.com/. https://formingworld.com/pareto-principle-forming-simulation/.
[08] Carleer, Bart. "The Big HOW of Setting Up a Process Simulation by Focusing on Essential Parameters: The AutoForm Pareto Principle Part Two." September. 2021. https://formingworld.com/. https://formingworld.com/pareto-principle-simulation-accuracy/.
[09] Carleer, Bart. „The Crucial Parameters: The AutoForm Pareto Principle Part Three.“ September 2021. https://formingworld.com/. https://formingworld.com/pareto-principle-crucial-parameters/.
[10] Carleer, Bart. „The Important Parameters: The AutoForm Pareto Principle Part Four.“ September 2021. https://formingworld.com/. https://formingworld.com/pareto-principle-important-parameters/.
[11] Carleer, Bart. „Focusing on Robustness: The AutoForm Pareto Principle Part Five.“ October 2021. https://formingworld.com/. https://formingworld.com/pareto-principle-robustness/.
[12] Carleer, Bart. „Tribology and Contact Pressure Parameters: The AutoForm Pareto Principle Part Six.“ 12. October 2021. https://formingworld.com/. https://formingworld.com/pareto-principle-tribology/.
[13] Schuler Pressen GmbH. „Visual Die Protection - Protecting Die, Preventing Damage.“ 2021. https://digitalsuite.schulergroup.com/en/digitalsuite/. https://digitalsuite.schulergroup.com/en/digitalsuite/visualdieprotection/index.html.
[14] International Organization for Standardization, ISO.org. „Quality Management Principles.“ kein Datum. https://www.iso.org/. https://www.iso.org/search.html?q=quality%20management.
[15] IEEE Computer Society. „Software Engineering Body of Knowledge (SWEBOK).“ kein Datum. https://www.computer.org/education/bodies-of-knowledge/software-engineering. https://www.computer.org/education/bodies-of-knowledge/software-engineering.
[16] The American Society of Mechanical Engineers. „Codes & Standards / Publications Information / Verification, Validation and Uncertainty Quantification.“ kein Datum. https://www.asme.org/codes-standards/publications-information/verification-validation-uncertainty. https://www.asme.org/codes-standards/publications-information/verification-validation-uncertainty.
[17] Carleer, Bart. "How Can You Prove Accuracy in Sheet Metal Simulation ? The Principle of the Accuracy Footprint Unveiled." May. 2020. https://formingworld.com/. https://formingworld.com/autoform-accuracy-footprint/.
[18] Schaeffler, Daniel. „Approach Stamping Problems Like a Crime Scene Investigator.“ April. 2021. MetalForming Magazine. https://www.metalformingmagazine.com/article/?/materials/high-strength-steel/approach-stamping-problems-like-a-crime-scene-investigator.
[19] Lee, Intaek. „See the Webinar Video: What is the Right Way to Understand Accuracy? A New Argument – A New Way of Thinking!“ 4. August 2020. https://formingworld.com/. https://formingworld.com/understanding-accuracy-video/.
[20] Krainer, Andreas. „Simulation-Based Inline Process Control: Franke Optimizes Production of a Kitchen Sink for Scrap Reduction of Over 50%.“ 24. November 2020. https://formingworld.com/. https://formingworld.com/simulation-based-inline-process-control-franke/.
[21] GROUP RENAULT. „GROUPE RENAULT “RENAULUTION” STRATEGIC PLAN.“ 14. January 2021. https://www.renaultgroup.com/. https://www.renaultgroup.com/wp-content/uploads/2021/01/groupe-renault-presse-release-renaulution-plan-14-january-2021.pdf.